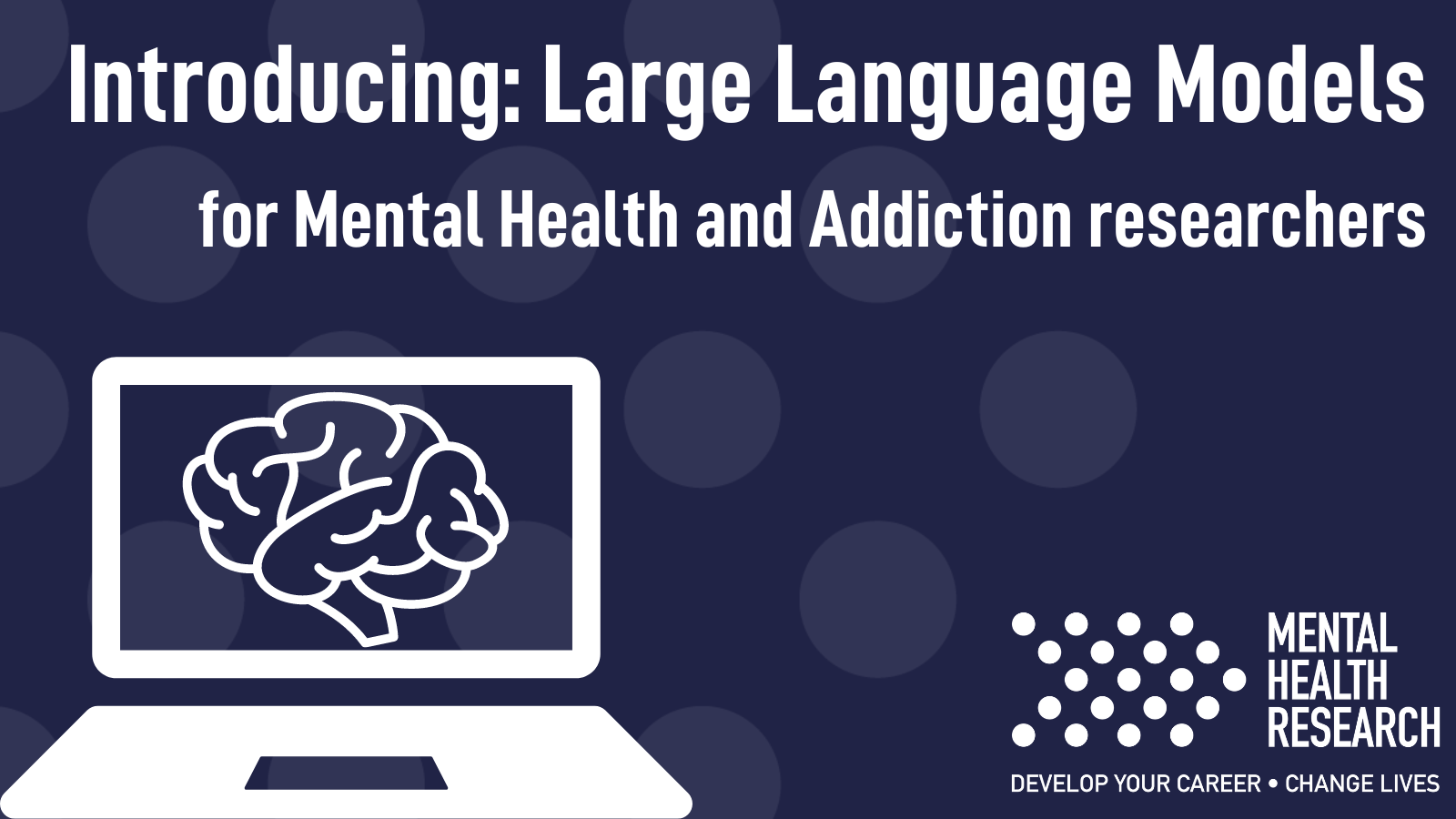
This article is intended as an introduction to large language models (LLMs) and how they might be of use to mental health and addiction researchers. The use of AI is increasing in all walks of life and has been used in various ways in health research for several years now. Their use and implementation will only to continue to grow in the coming years and so it’s worth getting to grips with them now.
If you are already familiar with LLMs then this might be a bit basic for you. However, if you have only heard of them in passing, never thought about them as research tools, or have no clue what I am talking about at all, read on!
What are LLMs?
The development of Large Language Models is one particular trend in AI that has garnered substantial attention. These are things such as OpenAI’s GPT (Generative Pre-trained Transformer) series, or Microsoft’s Copilot.
These models can generate text that is indistinguishable from that written by humans. LLMs are trained on diverse datasets, which contain a broad range of text from the internet.
By processing millions of documents, these models learn human language patterns, which enables them to predict the next word in a sentence with remarkable accuracy. Essentially, LLMs assimilate a vast amount of written material, which allows them to mimic the style and content of human authors.
In the last 12-18 months LLMs have taken massive leaps forward, particularly in terms of accuracy and usability. Context windows are significantly increased, meaning the amount of information the LLM can retain in a single conversation as reference material has got to the point where you can use it for some pretty advanced analysis, production or editing.
Use in research – the positives
In some research settings, LLMs are proving to be invaluable. They aid in literature reviews by summarising articles, generate research ideas based on current trends, and can even draft research proposals.
In fields with a lot of qualitative research, LLMs can analyse qualitative data and identify patterns in large datasets more quickly than human researchers. In psychiatry for example, LLMs are proving to be highly valuable in analysing brain scans. There are also multiple studies published recently which show LLMs are beginning to outperform doctors and medical professionals when writing up and summarising clinical notes, for example this one by Van Veen et al. With the concomitant rise of digital health records, this type of use could greatly streamline workloads for already stretched medical professionals.
Use in research – the areas for caution
While LLMs provide extensive capabilities, they also come with challenges.
A primary concern is their tendency to perpetuate biases found in their training data. Therefore, for researchers using LLMs, it’s crucial to be aware of the possibility of bias and to avoid reinforcing these biases in scientific work.
The integration of LLMs and AI in research also brings up ethical questions about authorship, data privacy, and the potential misuse of automated systems.
A specific example of data privacy issues is that unless you change the settings, data you put into ChatGPT can be used by OpenAI (the developer) in future training runs for the LLM. Therefore there is a chance that if someone else were to ask a question which required data or evidence that exactly matched yours, the system could rely on your data in forming its answer. It would almost certainly be out of context and at limited risk of plagiarism, however, it could violate ethics or GDPR rules for your research or institutional data regulations.
Privacy and data control is an area where academic institutions and research groups must develop guidelines to govern the responsible use of AI tools in research. Many already have and they will almost certainly be continually updated as the capabilities develop.
A further pitfall is the potential for error. ChatGPT is pretty powerful in what it can generate and is increasingly accurate in its output. However, it can still produce errors and make things up (termed hallucinations). Therefore, it is important that any work you use it to help with is something you understand how to do, or at least can check yourself.
Learning and resources
In preparation for this post I played around with ChatGPT and Copilot. The best way to learn how to work LLMs is to use them. I also took an intro course on LLMs for researchers offered by our IT department (University of Oxford) – many other institutions will almost certainly start offering these courses.
Copilot is included as part of Microsoft 365 and will probably be available to many researchers for free, so might be a good LLM to try out first. Coursera has a free course to help with the basics.
Conclusion
As AI and LLMs continue to evolve, they are likely to become more integrated into the fabric of academic research. These tools have immense potential to enhance productivity, foster innovative approaches to complex problems, and streamline data analysis.
However, it’s also imperative that the academic community stays informed about these technologies to ensure their use supports the integrity and advancement of science.
By responsibly embracing these technologies, researchers can unlock new potentials across disciplines, paving the way for significant advancements and breakthroughs in their respective fields.
This post was written with the aid of ChatGPT and Copilot.
What are your experiences with or thoughts on using LLMs as a researcher? Let us know over @MHRIncubator